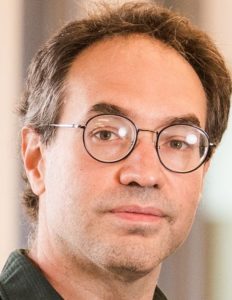
Ryan Baker
What is unique about your work on emerging technologies for teaching and learning?
I like to think that what makes our research group unique is our blend of data science and qualitative research. We use data science to develop models of constructs like engagement, self-regulated learning, affect, and complex student conceptual understanding. But these models are anchored in human understanding of these constructs, from methods like classroom observations, video replays, and text replays. And we use these models not only in purely data science analyses but also to support qualitative research, such as identifying critical events during learning in real-time, and notifying a classroom researcher so that they can conduct an immediate interview of the student to understand what just happened. We’re willing to share these tools with any interested researcher, by the way. Contact us!
What is your most recent insight from your project on emerging technologies for teaching and learning? What are 1-2 challenges you are facing?
We don’t have just one project—we partner with other researchers in academia and industry to help them answer their research and development questions. But one recent insight that I’m particularly enthusiastic about is evidence on how anxious and non-anxious students respond differently to frustration. In one of our studies, students developing concept maps of scientific phenomena behaved very differently when they became frustrated. Non-anxious students persisted in trying to figure out exactly what was incorrect and fix it, whereas anxious students deleted the whole concept map (including a lot of correct stuff!) and started over.
How have you attended to equity-related issues within your project? Please discuss actions taken.
Equity is extremely important to us. I’d like to share a finding from our recent review on algorithmic bias in education. We found evidence that algorithmic bias impacts many algorithms—educational algorithms function less effectively for historically underrepresented learners. In addition, we found that it occurs not just in terms of well-known demographic categories, but also in terms of a lot of under-studied categories such as urbanicity and native language. Our biggest takeaway is that the field doesn’t know what it doesn’t know—a wide range of identity variables seem to matter, but in general people aren’t even collecting those variables.
Read more:
- How Anxiety Affects Affect: A Quantitative Ethno- graphic Investigation using Affect Detectors and Data- Targeted Interviews.
Algorithmic Bias in Education - Comparing machine learning to knowledge engineering for student behavior modelling: A case study in gaming the system.
- Assessing Implicit Computational Thinking in Zoombinis Puzzle Gameplay
- Assessing Implicit Science Learning in Digital Games
- Improving Sensor-Free Affect Detection Using Deep Learning